Analysis of Adversarial Attacks on AI-based With Fast Gradient Sign Method
Keywords:
Adversarial, FGSM, Artificial intelligence, CleverHans, Mnist DatasetAbstract
Artificial intelligence (AI) has become a key driving force in sectors from transportation to healthcare, and is opening up tremendous opportunities for technological advancement. However, behind this promising potential, AI also presents serious security challenges. This article aims to investigate attacks on AI and security challenges that must be faced in the era of artificial intelligence, this research aims to simulate and test the security of AI systems due to adversarial attacks. We can use the Python programming language for this, using several libraries and tools. One that is very popular for testing the security of AI models is CleverHans, and by understanding those threats we can protect the positive developments of AI in the future. this research provides a thorough understanding of attacks in AI technology especially in neural networks and machine learning, and the security challenge we face is that adding a little interference to the input data causes the AI model to produce wrong predictions in adversarial attacks there is the FGSM model which with an epsilon value of 0.1 causes the model suffered a drastic reduction in accuracy of around 66%, which means that the attack managed to mislead the model and lead to incorrect predictions. in the future understanding this threat is the key to protecting the positive development of AI. With a thorough understanding of AI attacks and the security challenges we address, we can build a solid foundation to effectively address these threats.
Downloads
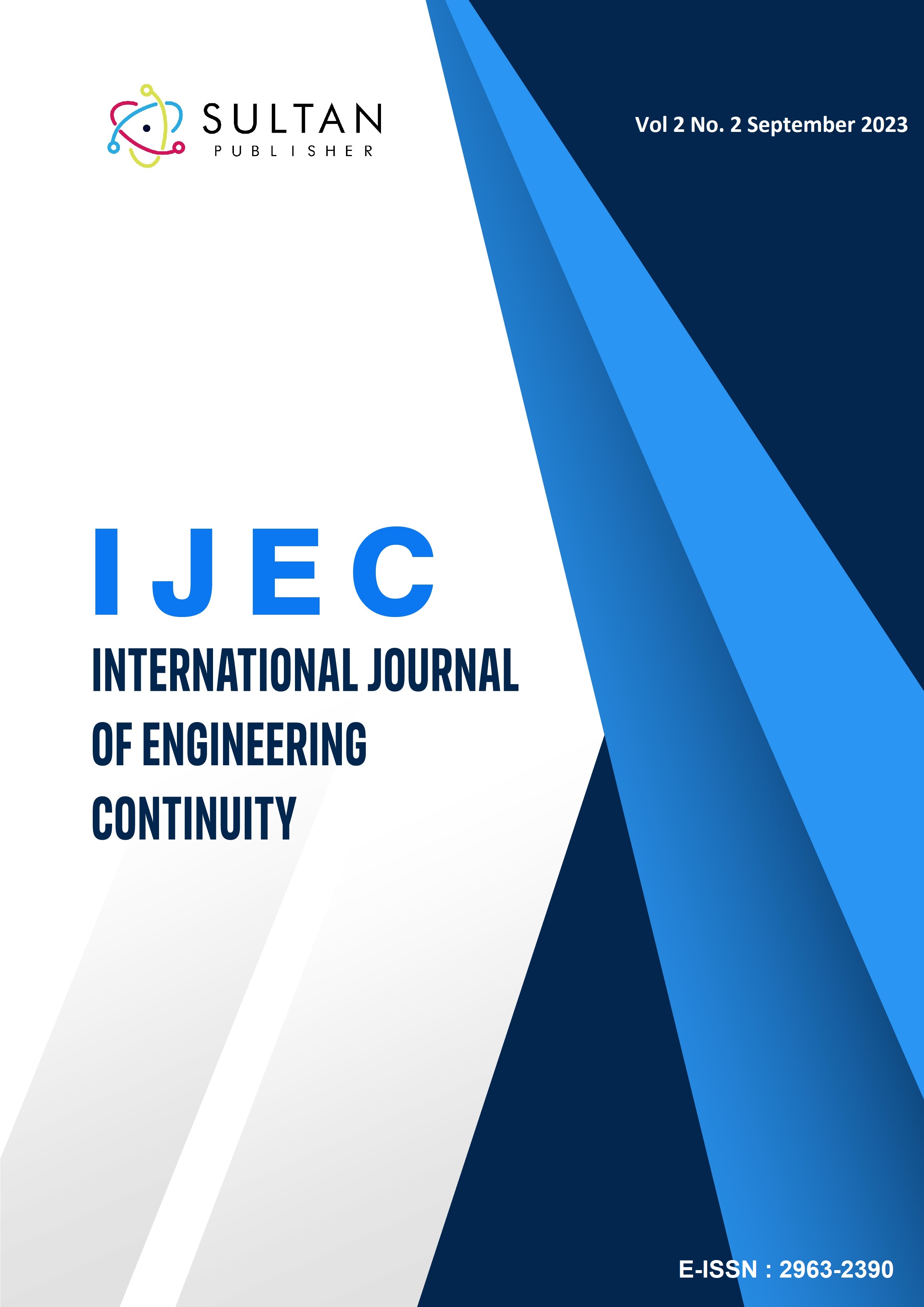
Published
How to Cite
Issue
Section
Copyright (c) 2023 SIGIT WIBAWA

This work is licensed under a Creative Commons Attribution-ShareAlike 4.0 International License.