SMOTE Variants and Random Forest Method: A Comprehensive Approach to Breast Cancer Classification
Keywords:
Breast Cancer Prediction, Machine Learning in Health, Smote Variants, Random ForestAbstract
This research focused on using machine learning methods for breast cancer diagnosis, considering that breast cancer is the scariest disease for women because it can cause mortality. Not only that, but there is also an increase in breast cancer death rates in women yearly. Early prediction is the right solution to increase life expectancy and reduce mortality rates caused by breast cancer. However, breast cancer data has a problem, namely that the data is imbalanced, which harms the performance of the machine learning method itself. In the data, breast cancer had a Benign class (357 instances) more than the Malignant class (212 instances). Therefore, this study aimed to solve the problem of imbalanced data using the Smote variants and Random Forest approaches in breast cancer classification. The results of this study showed that the Smote approach with Random Forest had the best performance compared to Borderline Smote and Random Forest in the case of breast cancer data classification, where Smote with Random Forest produced an accuracy of 97.3%, sensitivity of 96.9%, and specificity of 97.8%. In comparison, Borderline Smote with Random Forest produced an accuracy of 96.4%, sensitivity of 95.6%, and specificity of 96.9%. The results of this study can contribute to predicting breast cancer using the proposed method, because it has been proven to have high accuracy.
Downloads
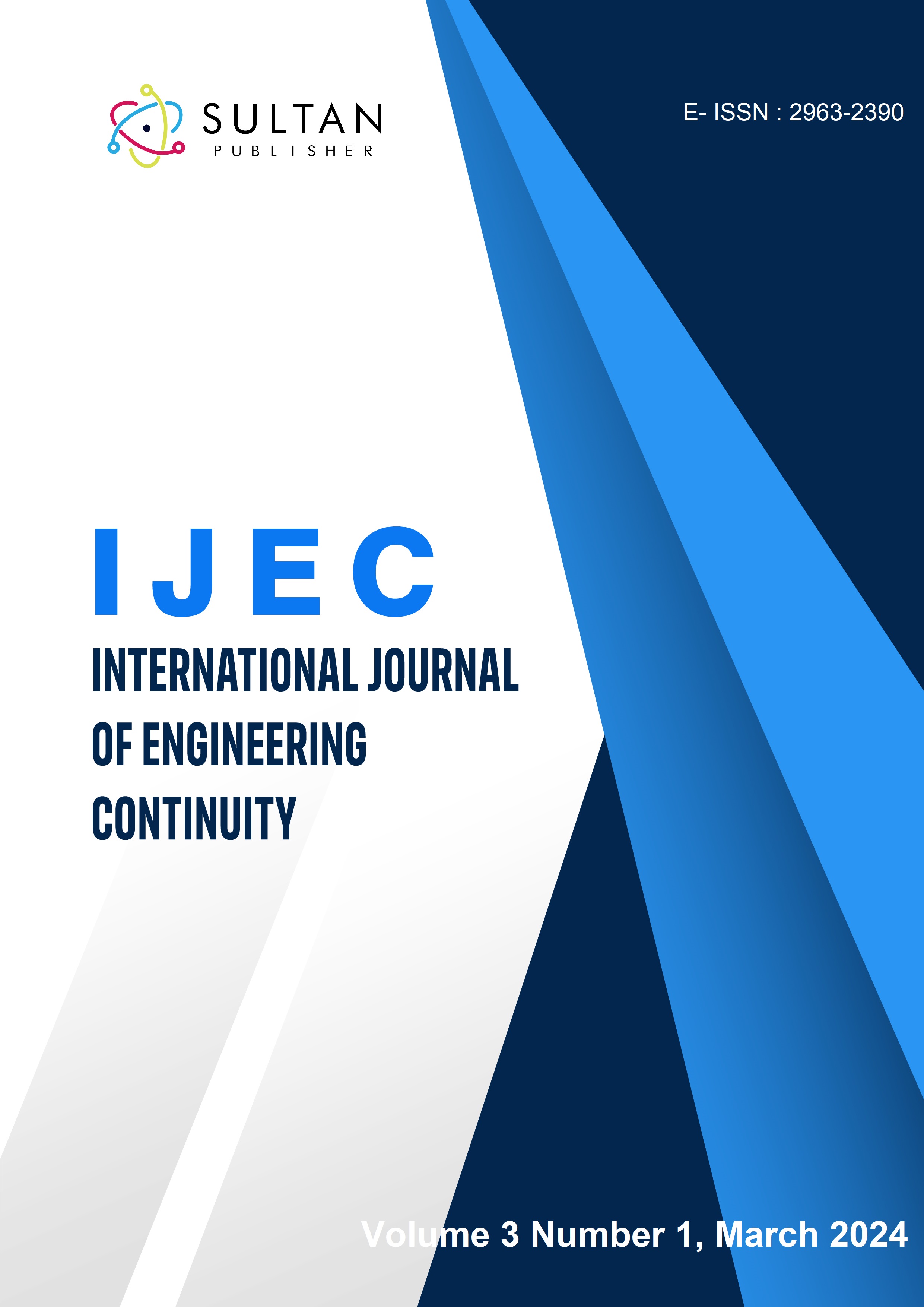
Published
How to Cite
Issue
Section
Copyright (c) 2024 Baiq Candra Herawati, Hairani Hairani, Juvinal Ximenes Guterres

This work is licensed under a Creative Commons Attribution-ShareAlike 4.0 International License.