Predicting Water Levels from Environmental Parameters Using Random Forest Models
Keywords:
Soft sensor, water level, Random Forest, atmospheric data, machine learningAbstract
Real-time monitoring of sea water levels is essential for maritime safety, coastal management, and disaster mitigation. This study addresses the challenges of sensor dependency and environmental vulnerability in traditional monitoring systems by proposing a machine-learning-based soft sensor. A Random Forest model was developed to predict sea water levels using atmospheric parameters such as barometric pressure, temperature, and relative humidity, leveraging data collected over seven months at one-minute intervals from a Marine Automatic Weather Station (AWS) in Tanjung Priok, Indonesia. Data preprocessing included outlier removal, normalization, and temporal feature extraction. The model achieved a high correlation coefficient (R = 0.8415) and low error metrics (MSE = 0.0209, RMSE = 0.1448), demonstrating robust predictive performance. The findings confirm the model's ability to capture tidal patterns and its potential to complement or replace physical sensors in harsh maritime environments. This research contributes to the field by improving monitoring resilience and reducing dependency on hardware sensors. Future work will explore integrating additional environmental variables, temporal modeling techniques, and hybrid approaches to further enhance prediction accuracy and robustness.
Downloads
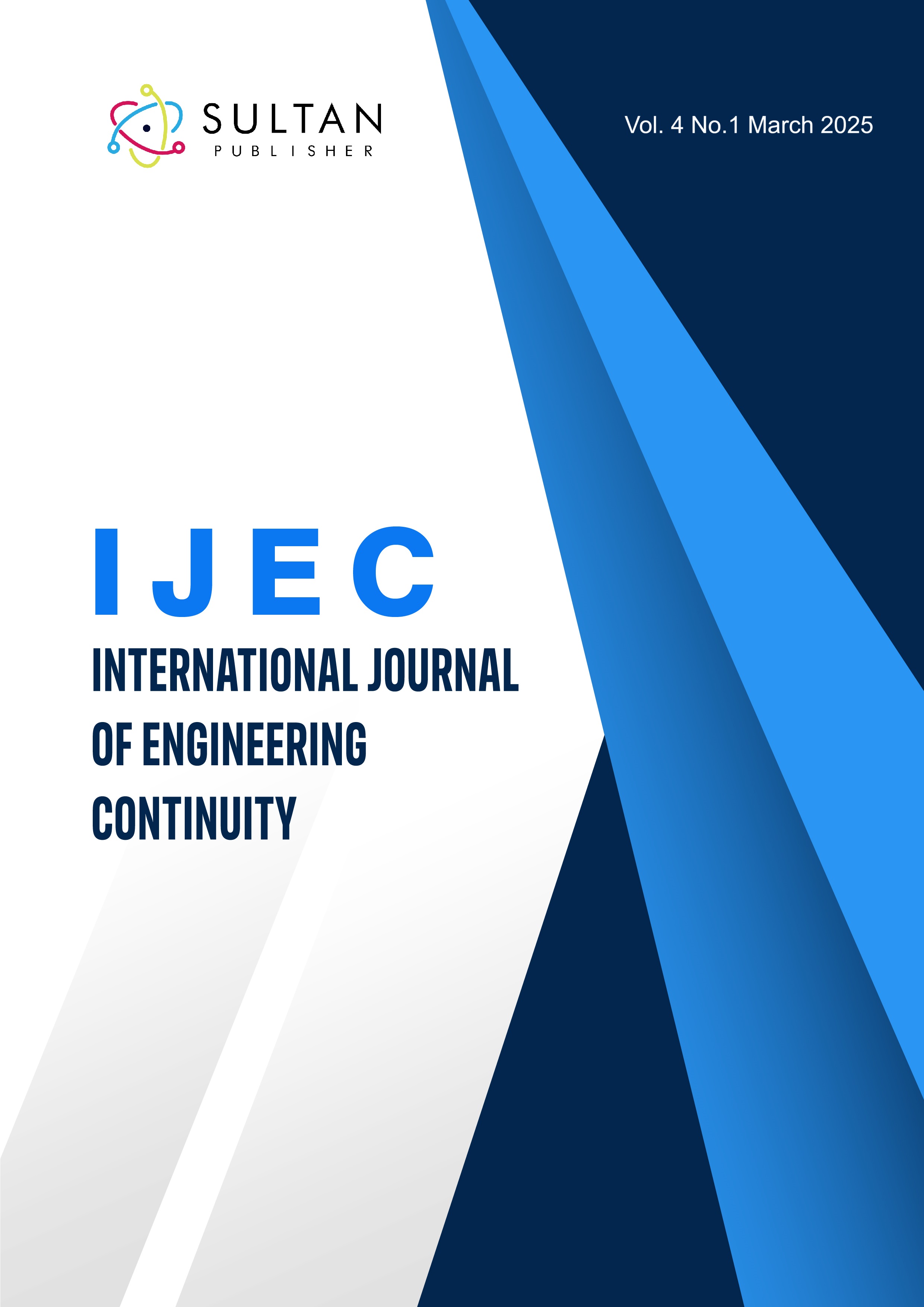
Published
How to Cite
Issue
Section
Copyright (c) 2025 Arum Putri Kusuma Anggraini, Trismadi Trismadi, Asep Adang Supriyadi

This work is licensed under a Creative Commons Attribution-ShareAlike 4.0 International License.