Ensemble Combination of CNN for MRI-Based Brain Tumor Classification
Keywords:
Ensemble Combination, Convolutional Neural Network, Brain Tumor ClassificationAbstract
Classifying 17 types of brain tumors remains a major challenge in the medical field, especially in improving diagnostic accuracy and accelerating patient care. This study proposes a CNN-based model with an ensemble combination approach to improve accuracy by integrating multiple architectures through Majority Voting and Weighted Average for more reliable predictions. The models are evaluated using accuracy, precision, recall, and F1-score metrics. The results show that CNN3 with Nadam achieves the best performance (accuracy: 0.90–0.91), outperforming CNN1 (0.87–0.89) and CNN2 (0.82–0.87). The ensemble combination improves accuracy across all models, with CNN3 achieving the highest accuracy (0.96), followed by CNN1 (0.94–0.95) and CNN2 (0.91–0.92). This study demonstrates that the ensemble combination approach can improve the performance of brain tumor classification using deep learning, contributing to faster and more accurate medical diagnosis. Furthermore, these findings open up opportunities for further research in advancing brain tumor detection systems.
Downloads
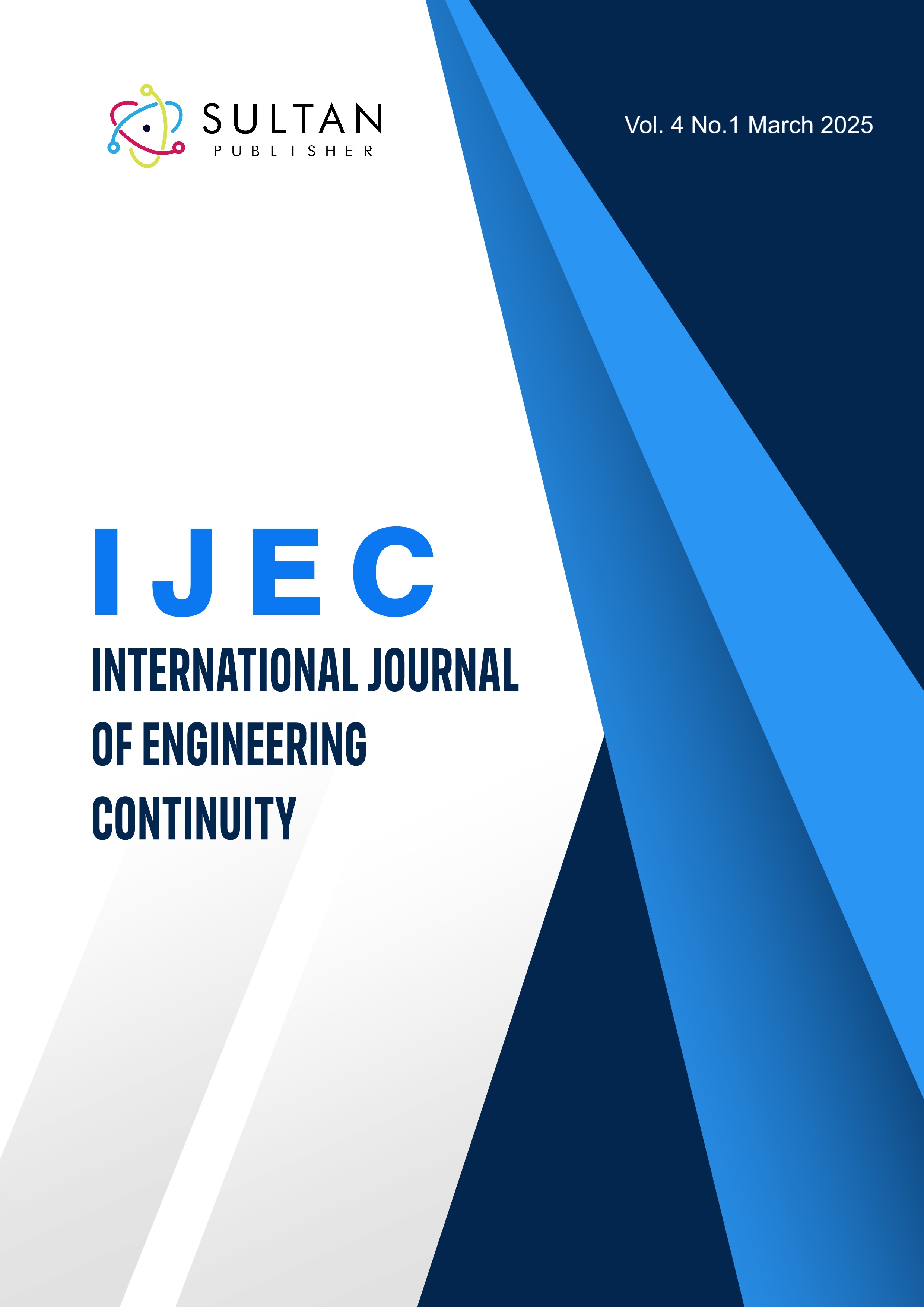
Published
How to Cite
Issue
Section
Copyright (c) 2025 Akbar Sidqi, Irwan Budi Santoso, Sri Harini

This work is licensed under a Creative Commons Attribution-ShareAlike 4.0 International License.